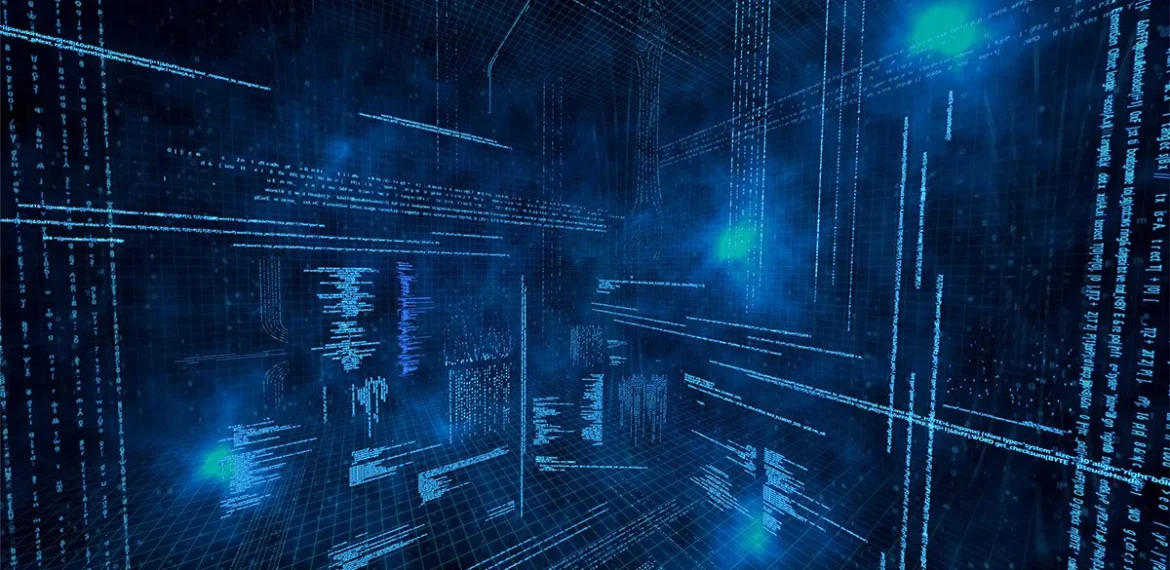
Synthetic Data Engineer In Chennai
Synthetic Data Engineer: Empowering the Future of AI and Machine Learning
In the world of artificial intelligence (AI) and machine learning (ML), high-quality data is the cornerstone of building powerful algorithms and models. However, obtaining vast amounts of real-world data can be expensive, time-consuming, or even impossible due to privacy and ethical concerns. This is where Synthetic Data Engineers come in—experts who specialize in creating realistic, AI-generated datasets that can be used for training and testing machine learning models.
At Sharaa Group, we understand that synthetic data is a transformative tool for businesses seeking to develop AI models while overcoming the challenges of real-world data limitations. In this blog post, we will explore the role of a Synthetic Data Engineer, the skills required, and why this position is crucial in today’s AI-driven world.
What is a Synthetic Data Engineer?
A Synthetic Data Engineer is a professional responsible for creating, managing, and refining synthetic datasets that are used to train, validate, and test machine learning models. Unlike real-world data, which may be hard to access or contain biases, synthetic data is generated using algorithms that replicate the statistical properties of real data, while ensuring privacy, security, and scalability.
Synthetic data can be used in a variety of applications, including autonomous vehicles, healthcare systems, financial forecasting, and more. These engineers focus on generating realistic data that can closely resemble actual user behaviors, sensor data, or environmental conditions, without the constraints that come with gathering real data.
Key Responsibilities of a Synthetic Data Engineer
Synthetic Data Engineers are responsible for a wide array of tasks that ensure data quality and model accuracy. Here are some of their primary responsibilities:
-
Data Generation and Simulation:
Synthetic Data Engineers develop algorithms and models that generate realistic data, simulating real-world scenarios for machine learning tasks. This includes using techniques such as generative adversarial networks (GANs), data augmentation, and simulated environments to produce diverse datasets. -
Creating Datasets for Specific Use Cases:
These engineers work with domain experts to create synthetic datasets that meet specific project requirements. For example, in autonomous driving, engineers might create synthetic datasets that simulate different driving conditions, traffic patterns, or pedestrian behaviors to train self-driving algorithms. -
Ensuring Data Realism:
One of the key challenges in synthetic data generation is ensuring that the data closely mirrors real-world characteristics. Synthetic Data Engineers must fine-tune the algorithms to capture real-world variability, noise, and edge cases, so that models trained on synthetic data are accurate and generalizable. -
Testing and Validating Models:
Engineers use synthetic data to validate machine learning models, especially when access to real-world data is limited or when data privacy concerns arise. They test models on these datasets to ensure they perform accurately before deploying them in real-world applications. -
Data Augmentation:
Synthetic data can also be used for data augmentation—creating variations of real data to improve model performance. By introducing slight modifications to existing data, engineers can generate new data points that help models become more robust and better at generalizing to unseen examples. -
Managing and Maintaining Data Pipelines:
Synthetic Data Engineers work on building and maintaining data pipelines to ensure that synthetic data is seamlessly integrated into machine learning workflows. This may involve automating data generation, cleaning, and validation processes to ensure data is ready for use by data scientists and engineers. -
Collaboration with Data Scientists and Engineers:
Synthetic Data Engineers collaborate closely with data scientists, machine learning engineers, and domain experts to understand the requirements for synthetic data and ensure it is generated and used appropriately. They provide valuable insights into how data can be leveraged for training and testing AI models. -
Ethical Considerations and Privacy Concerns:
One of the advantages of synthetic data is that it can be generated without violating privacy rights or exposing sensitive information. Engineers must consider the ethical implications of synthetic data and ensure that the generated datasets are compliant with data privacy laws and regulations such as GDPR or HIPAA.
Skills Required to Become a Synthetic Data Engineer
The role of a Synthetic Data Engineer requires a combination of technical, mathematical, and domain-specific knowledge. Here are some essential skills for this role:
-
Strong Programming Skills:
Synthetic Data Engineers need to be proficient in programming languages such as Python, R, Java, or C++, and familiar with machine learning libraries like TensorFlow, PyTorch, or scikit-learn. -
Understanding of Machine Learning and AI:
These engineers must have a strong understanding of machine learning concepts, algorithms, and models. Knowledge of deep learning techniques, such as GANs, is especially valuable for generating high-quality synthetic data. -
Experience with Data Simulation:
Familiarity with simulation tools and frameworks for generating synthetic data is essential. This may include using simulated environments (e.g., Unity, CARLA for autonomous vehicles) or specialized tools for specific industries. -
Statistical and Mathematical Expertise:
Synthetic Data Engineers need a solid understanding of statistics and probability to ensure that the generated data is realistic and representative of real-world conditions. Knowledge of data distributions, noise modeling, and bias correction is critical. -
Data Engineering and Pipeline Development:
Experience in building and maintaining data pipelines, as well as automating data collection and processing, is crucial for efficiently generating and managing synthetic datasets. -
Knowledge of Data Privacy and Ethics:
Engineers must be familiar with data privacy regulations and ethical considerations surrounding synthetic data. They should be able to generate data that is both useful and compliant with privacy laws. -
Problem-Solving and Critical Thinking:
Developing synthetic data that closely mimics real-world scenarios is a challenging task. Engineers need strong problem-solving skills to handle edge cases, validate data, and fine-tune models to improve data realism. -
Domain-Specific Expertise:
Depending on the industry (e.g., healthcare, autonomous driving, finance), having domain knowledge is important for ensuring that synthetic data accurately reflects real-world conditions in that field.
Why Synthetic Data Engineers Are Crucial for the Future
Synthetic data is a game-changer for industries that rely on machine learning and AI. Here's why Synthetic Data Engineers are becoming increasingly important:
-
Overcoming Data Privacy Issues:
In industries like healthcare and finance, data privacy is a major concern. Synthetic data can be generated without compromising sensitive personal information, allowing AI models to be trained without violating privacy regulations. -
Enabling Scalable Data Generation:
Real-world data collection can be time-consuming and costly. Synthetic Data Engineers make it possible to generate large amounts of high-quality data in a fraction of the time, helping companies scale AI development quickly. -
Creating Diverse Datasets:
Real-world data is often limited or biased. By generating synthetic data, engineers can ensure that datasets are more diverse, balanced, and inclusive, which helps to build fairer and more accurate machine learning models. -
Accelerating Model Development:
With synthetic data, machine learning models can be tested and trained faster, reducing the time-to-market for new AI products and services. This is particularly important in industries like autonomous vehicles or robotics, where real-world testing can be expensive and dangerous. -
Supporting Innovation in High-Risk Industries:
In sectors like healthcare, aerospace, and defense, real-world testing of AI models can be risky or impractical. Synthetic data allows these industries to test and refine models without the risk of failure in real-world environments.
Applications of Synthetic Data Engineering
Synthetic Data Engineers play a vital role in several industries, including:
-
Autonomous Vehicles:
Creating synthetic driving data to train self-driving algorithms in various road conditions, weather, and traffic patterns. -
Healthcare:
Generating medical imaging data or patient health data to train models for diagnostic AI without compromising patient privacy. -
Finance:
Simulating financial data to train fraud detection algorithms and risk models without using sensitive customer data. -
Retail:
Generating customer behavior data to improve recommendation engines, sales forecasting, and inventory management. -
Robotics:
Simulating environmental data for robot training, enabling robots to interact safely and efficiently in a wide variety of settings.
Conclusion
Synthetic Data Engineers are pioneering a new era of AI development by enabling the creation of vast, realistic datasets that overcome the challenges of real-world data limitations. Their work empowers businesses to build smarter, more efficient AI models, while ensuring privacy and compliance with data regulations. At Sharaa Group, we recognize the power of synthetic data in driving AI innovation, and we are committed to helping companies harness this technology to unlock the full potential of artificial intelligence.